Build Vs Buy Generative AI - All You Need To Know In 2025
Get to know the deciding factors on Building Vs Buying Generative AI solution for your business, whether to build your own AI or buy one.
- Jan 13 2024
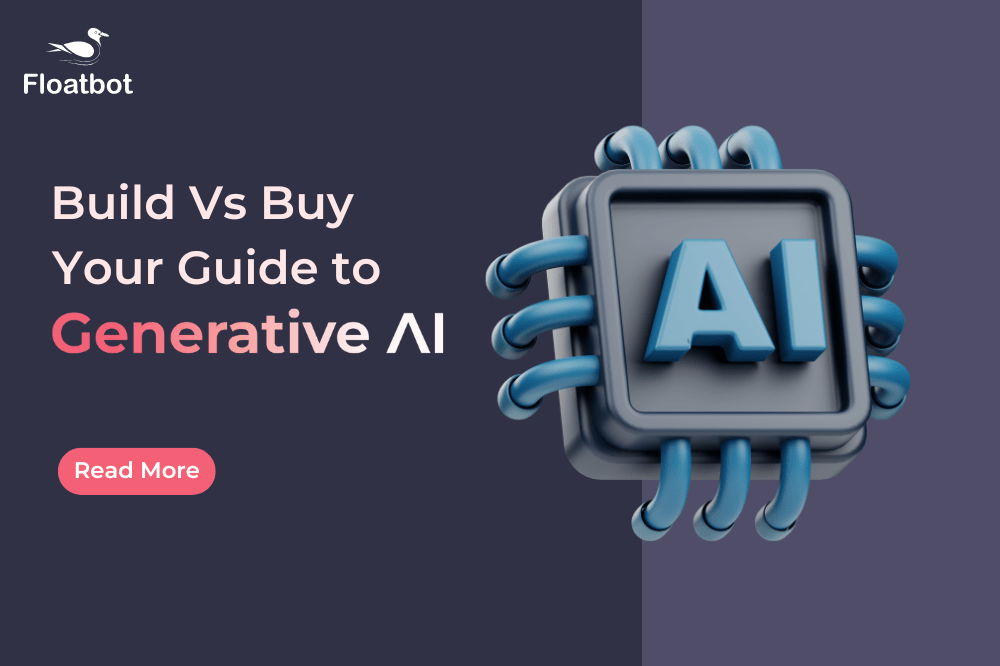
Prologue
As the age of artificial intelligence (AI) dawns, businesses worldwide have acknowledged that they risk falling behind the growth curve if they fail to leverage its potential. Organizations will inevitably adopt AI that generates texts, images, audio, videos, or a mix of these. However, as they explore transformative technology, addressing the dilemma of build vs buy generative AI comes to the forefront. With a wide range of options available for customizing off-the-shelf AI services vis-a-vis the significant effort needed to build a proprietary generative AI from scratch, the decision is never an easy one. This blog will probe both sides of the dilemma to help you put your best foot forward.
Thinking Strategically While Implementing GenAI
According to Gartner, GenAI is increasingly speeding up modernization efforts and by 2027, GenAI tools will revitalize legacy business applications and develop appropriate replacements, slashing modernization costs by 70%. However, AI (GenAI) services are unlike any other IT service, they require input from cross-functional teams - ranging from data analysts to product owners to thrive.
Business leaders must think strategically and include all the stakeholders and potential users in the decision-making process. This will inadvertently figure out the features and functionalities that are priorities for the AI-based solution. Needless to say, one of the most critical aspects is how the business operatives interact and blend with the AI solution. From managers to frontline agents to analysts - everyone must participate in the AI feedback loop. For instance, an insurance company manager might tap into the data that reveals customer sentiment to decide whether or not an AI bot requires extra training. Simultaneously a frontline agent might conduct a live conversation assisted by AI to retrieve data faster and offer immaculate resolutions quickly.
While internal data scientists can help to create your own AI model, taking it to production and implementing the model is a whole different story and requires niche expertise. At the same time, sticking to a projected timeline to implement a GenAI project is equally important. So, are you willing to afford the wait to build an AI from scratch?
In short,
- If you wish to advance and cement your competitive advantage backed by a strong data science team, build.
- If you are scouting for a niche solution and have limited capability and timeframe, buy.
Having said that, here is an interesting find from McKinsey. Did you know that the best performers in generative AI efforts are in fact less inclined towards cost reduction, a top priority for AI laggards? In fact, the top objective of GenAI high performers is to create new streams of revenue!
Build Vs Buy Generative AI - Weighing All The Critical Factors
1. Calculating The Cost Of AI Solution
The cost of ownership is an important consideration when deploying enterprise artificial intelligence (AI) solutions. If you are looking to build your own AI solution, the cost of computing equipment coupled with the recruitment of experienced developers and AI experts can burn a hole in your pocket. Additionally, businesses must budget for the long-term maintenance of an in-house corporate AI system. On the other hand, ready-made AI solutions are cost-effective since businesses simply have to make a one-time purchase or subscribe to an AI service on a pay-as-you-go approach. The AI vendor takes care of R&D, maintenance, and user support.
2. How Easy Is It To Integrate With Existing Digital Infrastructure?
An enterprise that needs the GenAI solution to interact efficiently with its existing digital infrastructure may opt to build it in-house. Establishing a proprietary GenAI solution allows a company to tailor their development strategy aligned with the requirements of existing software and infrastructure. In the long term, absolute control over customized AI functionalities can be expensive but worth the effort.
Alternatively, an organization planning to buy an AI solution must shortlist vendors capable of integrating an AI solution (mostly generative AI LLMs) with the pre-existing infrastructure. The best part is that it alleviates extensive fine-tuning, minimizing the time and effort needed to deliver excellent results. Rarely, you might encounter a vendor offering fantastic AI solutions that require time for integration. In such cases, it's always wise to choose quality over speed of deployment.
3. Identifying Regulatory Concerns
As the world becomes more and more data-driven, governments are tightening the noose on data with regulations that do not have transnational uniformity. Industries like healthcare and finance (and of course government organizations) are heavily regulated. Some regulations even mandate to keep sensitive data on-premises, which could be difficult to do with an off-the-shelf AI solution. As such, it is natural for businesses in these industries to consider developing their pet GenAI projects that guarantee compliance with regulations.
Nevertheless, businesses operating outside the strict regulatory overwatch can store data on the cloud. They can opt for GenAI services from a vendor since this approach wins on the affordability front.
4. Deployment Time
A business must estimate when it needs the enterprise GenAI solution to be ready to go. If the leadership feels that it has the patience to wait a couple of months or years with a large funding, it can design its own AI system. However, in today's competitive landscape, many startups often require a GenAI solution in a matter of weeks. The best course of action for them is to purchase a GenAI solution from a vendor. During the planning stage, company executives must thoroughly define the project's goals and objectives, which will help them to determine when an AI-powered solution will be required.
Key Recommendations While Developing Or Testing GenAI Solutions
Orthodox approaches to solution design are becoming redundant as the world evolves from linear to open design principles with genAI solutions. For a long time, the user experience and customer journey have been defined by the marketing experts in a not-so-personalized linear fashion. The same logic percolated to traditional chatbots that limited the bot to handle unstructured requests.
However, generative AI solutions have redefined the relationship with users and have become more non-linear. For any interaction with a bot to feel realistic, the conversations must be open and free. This is exactly where ChatGPT and Bard have succeeded and took the world by storm. By mimicking human conversations and developing an essence of freedom, they excel at natural interactions . Nevertheless, this newfound freedom comes with design challenges to develop applications around genAI. Even GenAI systems require an integration and deployment pipeline. Also, they need to be maintained after deployment.
Here are some pivotal recommendations while developing or testing GenAI solutions
1. Demonstrate Value By Showcasing Extra Features
Users are never aware of the ultimate capabilities of GenAI solution. Allowing the AI to demonstrate its value by showcasing extra features beyond a user's initial query can be beneficial. It is possible to communicate these capabilities in various formats, like on the landing pages of the solutions, through pop-ups around the chat window, or even in a live chat where the AI highlights its capabilities.
2. Quickly Captivate Users
Traditional customer service depends on assembling detailed information via user inputs. For example, while ordering food, an app validates data on location, restaurant, cuisine, food items, personalized delivery instructions, payment information and what not. In contrast, genAI allows for a natural dialogue and captivates user interest by delivering value at the very start of an interaction. It can offer recommendations upfront (by retrieving data on customers in the background) and progress to answer any query/request through a free-flowing conversation.
3. AI-led Interaction By Pre-empting Behavior Patterns
GenAI has the capacity to pivot the interaction dynamic from a simple question-and-response conversation to a more proactive approach with follow-up questions by pre-empting behavior patterns or conversation context. This shift dynamic unlocks possibilities for deeper and meaningful customer relationships and engagement enabling businesses to align with customer intent.
4. Relationship & Retention
With GenAI, each chat session can be considered a building block in a relationship, with new sessions building upon previous ones to deepen the understanding of user requirements. It opens the door to a high degree of personalization, which in turn, boosts loyalty and retention. By leveraging generative AI's memory capacities, a business can enhance its recommendation engine, making it difficult for users who would not wish to forfeit benefits accumulated by having a long conversation history.
Closing Thoughts
The deployment of generative AI marks a paradigm shift in the way businesses operate. Organizations must address the build vs buy generative AI dilemma by carefully considering all the relevant end goals - boosting competitiveness, optimizing operations, driving innovation, creating new revenue streams, etc. All of it hinges on a thorough investigation of business-specific specific needs, competencies, and strategic objectives. If you are looking to talk to a GenAI expert to guide you, the wait ends here! Give us a call today to learn more about GenAI deployment that matches your criteria.